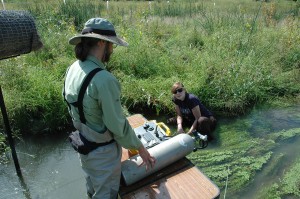
Students measure the critical shear stress of sediment entrainment under a submersed vegetation patch, Big Spring Run, PA.
The approach that we take to understanding the dynamics of physical-biological interactions in ecosystems and their long-term effects in the Environmental Systems Dynamics Laboratory (ESDL) mixes experimental and observational science with numerical modeling. The typical approach begins with a set of experimental or observational studies to understand hydroecological processes, typically at a small scale. Previous process-based studies have, for example, focused on elucidating the functional form of the physics of flow and sediment entrainment through arrays of vegetation. Other process-based studies may seek statistical associations between variables to develop a conceptual model about how those variables interact. The statistical techniques that we use need to be able to detect strongly nonlinear associations between multiple variables and variable types and are often fairly new to environmental science applications. Once the details of the processes are sufficiently understood, the next step is often to construct a simulation model to evaluate the impact of those processes over much longer timescales or broader spatial scales.
We often choose reduced-complexity modeling strategies to deal with the need to evaluate the impacts of physical-biological interactions over the spatial scale of landscapes and temporal scale of millennia. Somewhat of a misnomer, well-formulated reduced-complexity models should capture the complexity of interest (i.e. emergent phenomena) but with key simplifications of the physics. For instance, the reduced-complexity models that we typically use may reduce the dimensionality of the problem, solve approximations of governing equations, or employ rule-based rather than equation-based techniques. The assumptions or simplifications that we use in the models are then subject to rigorous validation and testing against field observations. Hence, our numerical modeling work and field/experimental work proceed in tandem.
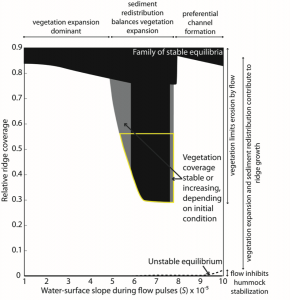
Results of a sensitivity analysis performed with a reduced-complexity model. The yellow region outlines the environmental conditions that can produce ridge-and-slough landscapes (see figure below). Click on the image to read more.
Once a simulation model is established and field-validated, it is then often perturbed in order to understand the sensitivity of some emergent phenomenon (e.g., landscape patterning) to changes in environmental drivers or to generate or test hypotheses about alternate mechanisms that could produce the emergent phenomenon. This phase of our approach to problems is also closely linked to field work. For example, modeling experiments may suggest that the type of landscape degradation observed in a particular location could arise from two different drivers (e.g., warmer temperatures, enhanced rainfall), but that one of those drivers produces a distinct pattern in another environmental variable (e.g., soil moisture), while the other produces a different pattern. This outcome would direct additional field work to reveal which perturbation was the likely driver of landscape degradation in that location.
A final use of models in the ESDL is to achieve a geographically broad, general understanding of the linkage between landscape pattern and process. We are finding, for instance, that some types of landscape pattern are indicative of specific driving processes such that insight gained about that landscape’s sensitivities and critical points in one location are transferable to another. Understanding similarities and universalities in the dynamical features of landscapes will reduce the need for intensive and costly data gathering for diagnosing environmental health problems or identifying susceptibilities to climate or land-use change.